Unlocking Efficiency: The Role of Machine Learning Data Labeling in Business
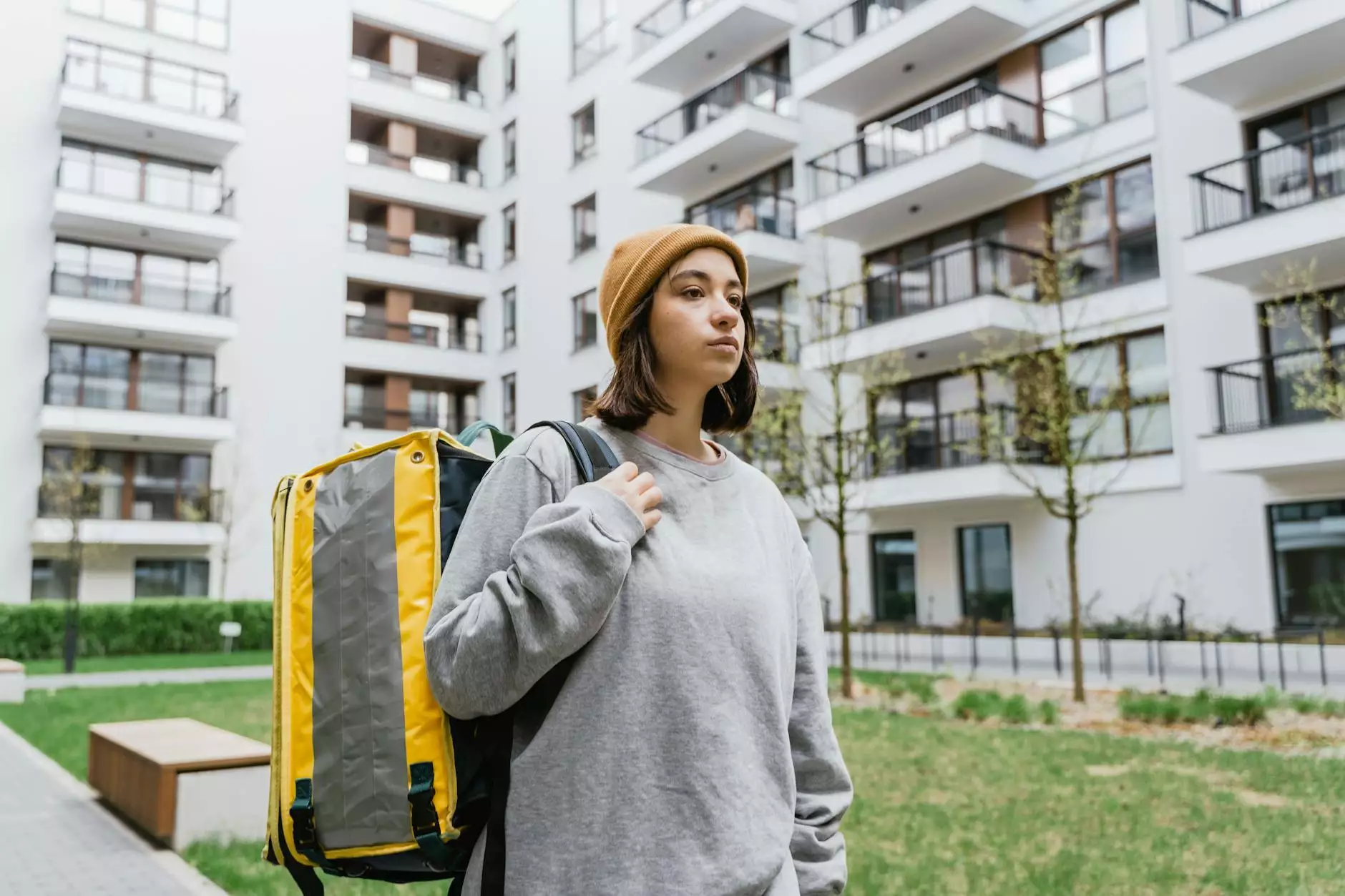
In today's fast-paced digital world, businesses are constantly seeking ways to enhance their operational efficiency and improve customer satisfaction. One technology that has proven invaluable in recent years is machine learning, an area of artificial intelligence that enables computers to learn from data and make decisions. A crucial component of machine learning is data labeling, an essential process that underpins the development of accurate machine learning models. This article delves into the significance of machine learning data labeling in various business contexts, focusing on home services and locksmith industries.
Understanding Machine Learning and Data Labeling
Machine learning refers to algorithms and statistical models that enable computers to perform specific tasks without explicit instructions, relying instead on patterns and inference. For machines to learn effectively, they must be trained on large datasets, which is where data labeling comes into play. Data labeling involves annotating data to create a training set that provides the necessary context for the machine learning models.
What Is Data Labeling?
Data labeling is the process of tagging or annotating data—whether images, text, audio, or video—so that a machine learning model can recognize patterns and make decisions based on that data. It can involve a variety of tasks, including:
- Image Annotation: Labeling images with relevant tags or bounding boxes.
- Text Labeling: Identifying parts of speech, sentiments, or specific entities in textual data.
- Audio Annotation: Tagging segments of audio files for speech recognition or sound classification.
- Video Annotation: Marking frames in a video for object detection and tracking.
The Importance of Quality Data Labeling
The success of a machine learning model heavily depends on the quality of the labeled data. High-quality data labeling ensures accurate training, leading to reliable predictions and classifications. Businesses must prioritize the following aspects when implementing data labeling:
- Consistency: Consistent labeling across datasets to avoid confusion during training.
- Accuracy: Precise annotations that accurately reflect the underlying data.
- Scalability: Ability to manage large volumes of data while maintaining quality.
- Relevance: Ensuring that labeled data is applicable to the specific machine learning task.
Applications of Machine Learning Data Labeling in Business
In various industries, including home services and locksmiths, the impact of machine learning data labeling is transformative. Here are some notable applications:
1. Home Services Industry
The home services industry encompasses a wide range of services, including plumbing, electrical work, and HVAC repair. By leveraging machine learning data labeling, businesses in this sector can enhance their operational efficiency and customer experience in various ways:
Enhanced Customer Support
AI chatbots powered by machine learning can analyze customer inquiries and provide instant support. Data labeling allows these systems to classify and respond to requests accurately, reducing response time and improving service speed.
Predictive Maintenance
With the help of labeled data from past service requests and equipment failures, companies can develop predictive maintenance models. This allows them to anticipate when maintenance is needed, thereby preventing costly breakdowns and ensuring customer satisfaction.
Optimized Scheduling
Machine learning models can analyze historical data on service requests to optimize scheduling for technicians. This results in more efficient use of resources and shorter wait times for customers.
2. Locksmith Industry
Similarly, the locksmith industry can benefit immensely from machine learning data labeling. Here’s how:
Fraud Detection
With data labeling, systems can identify patterns associated with fraudulent requests or potential security risks. By analyzing past locksmith calls, businesses can train models to flag suspicious behavior, thereby enhancing service security.
Inventory Management
Data labeling can be utilized to categorize and track inventory levels effectively. Machine learning models can predict the demand for various locking mechanisms based on historical data, enabling locksmiths to maintain optimal stock levels.
Smart Lock Solutions
As smart locks become increasingly popular, locksmiths can utilize labeled data to train systems for identifying and resolving compatibility or functionality issues, thus providing superior service to tech-savvy customers.
Implementing Machine Learning Data Labeling
For businesses looking to implement machine learning data labeling, it’s crucial to follow a structured approach:
Step 1: Define Objectives
The first step is identifying the goals of the data labeling project. Understand what specific problems you wish to solve and how labeled data will contribute to achieving those objectives.
Step 2: Choose the Right Tools
Various tools are available for data labeling, including open-source software and commercial platforms. Select tools that meet your team's skill set and project needs.
Step 3: Develop a Labeling Guide
Creating a labeling guide ensures consistency across your data labeling efforts. This guide should include examples, definitions, and instructions tailored to your specific needs.
Step 4: Train the Labelers
If you have a team conducting the labeling, invest time in training them. Familiarizing them with the labeling guide and letting them understand the objectives is essential for success.
Step 5: Conduct Quality Checks
Regularly review labeled data to maintain high standards. Implement quality control measures such as peer reviews or audits to ensure that the quality of data continues to meet your expectations.
The Future of Machine Learning Data Labeling in Business
The future of machine learning data labeling is bright, with advancements in automation and artificial intelligence. As businesses increasingly rely on data-driven decision-making, the demand for high-quality labeled data will continue to grow.
Trends to Watch
- Automated Data Labeling: Technologies that automate labeling tasks are developing rapidly, reducing the workload on human annotators and speeding up the labeling process.
- Human-in-the-Loop Systems: Combining human oversight with machine learning processes ensures that the labeled data is not only created quickly but also retains a high level of quality.
- Customization: Tailored labeling solutions that cater to specific industries, such as home services and locksmiths, are likely to emerge, offering enhanced relevance for businesses.
- Integration with Real-Time Data: The ability to label and learn from data in real-time will allow businesses to keep pace with ever-changing market demands and customer preferences.
Conclusion
As businesses navigate the complexities of the digital era, the integration of machine learning data labeling into operational strategies has become essential. It not only enables enhanced customer support but also drives predictive maintenance analytics, fraud detection, and intelligent inventory management. The ability to leverage data effectively will ultimately set businesses apart in a competitive marketplace. As the demand for accuracy and efficiency rises, mastering the art of data labeling will be key to unlocking the future success of businesses in the home services and locksmith sectors.
Investing in quality machine learning data labeling practices today holds the promise of a streamlined, customer-focused tomorrow.